Sports
Ziye Wang
For many basketball players, the NBA draft combine is a crucial stepping stone towards fulfilling a lifelong dream.
While it holds somewhat less weight for highly touted prospects, putting up big numbers on athletic tests like the max vertical jump or the three-quarter court sprint can be the difference maker between being picked up late in the second round or ending up overseas. For players looking to eke out a career in the best league in the world, performance at the combine is a needle mover.
That said, there’s a lot of discourse out there on just how much impact (or lack thereof) draft combine measurements actually have on a player’s success. After all, Kevin Durant famously couldn’t put up a single rep of 185 pounds on the bench and ended up being one of the greatest scorers in history.
The Slim Reaper notwithstanding, it’s clear that decision-makers across the league continue to place emphasis on the combine, what with the recent news that participation will now be mandatory for all invited prospects.
The question is—should they? Does raw speed, strength, and jumping ability actually translate to production at the NBA level? Let’s find out.
Data Collection
NBA draft combine metrics
To assess the predictive value of draft combine metrics on NBA success, we used Python to scrape draft combine results using the NBA’s official API, collecting all available data from 2000 to 2023. We narrowed down our metrics to the ones with the greatest amount of available data:
max vertical leap
lane agility time
three-quarter court sprint
bench press
We also wanted to consider anthropomorphic measurements (e.g., wingspan and weight), but since those are confounded by height, we calculated wingspan and weight ratio variables instead—that is, wingspan and weight divided by height. The greater an individual’s ratio is, the greater their wingspan or weight is relative to their height.
NBA success: LEBRON
The next part was a bit trickier: defining “NBA success”. There are countless metrics and stats one could use to go about operationalizing this admittedly vague term. For the sake of simplicity, we figured that an all-in-one advanced metric was the most reasonable. But even then, there are a bunch of options—from John Hollinger’s classic PER to FiveThirtyEight’s (now retired) RAPTOR model.
We decided to go for the new kid on the block—BBall Index’s LEBRON. Introduced in 2022, LEBRON “evaluates a player’s contributions using the box score [...] and advanced on/off calculations [...] for a holistic evaluation of player impact per 100 possessions on-court.” A detailed breakdown of how it’s calculated is beyond the scope of this article, but you can read more about it here. In essence, it’s an all-in-one metric for how impactful an NBA player is. It’s further broken down into Offensive LEBRON and Defensive LEBRON, so we ended up using all three in our analyses.
Using R, we scraped all LEBRON data from BBall Index (which goes back to the 2009 season), then computed career averages for LEBRON, O-LEBRON and D-LEBRON for every player (LEBRON is calculated per season). We then linked up this dataset with the NBA draft combine data we scraped earlier, leaving us with a final dataset of 924 rows (players) with LEBRON metrics and at least a few combine metrics. You can download this final dataset here. The raw LEBRON dataset is also available here.
Analyses & Results
The association between NBA draft combine metrics and LEBRON
We first ran linear regression analyses to assess the association between our 6 combine metrics (max vertical leap, lane agility time, three-quarter court sprint, bench press, wingspan ratio and weight ratio) and our three LEBRON metrics.
Here are the scatterplots of our findings, with statistically significant associations (after adjusting for multiple comparisons) highlighted in red. We standardized our combine metrics prior to analysis, which allows us to compare effect sizes across metrics with different units.
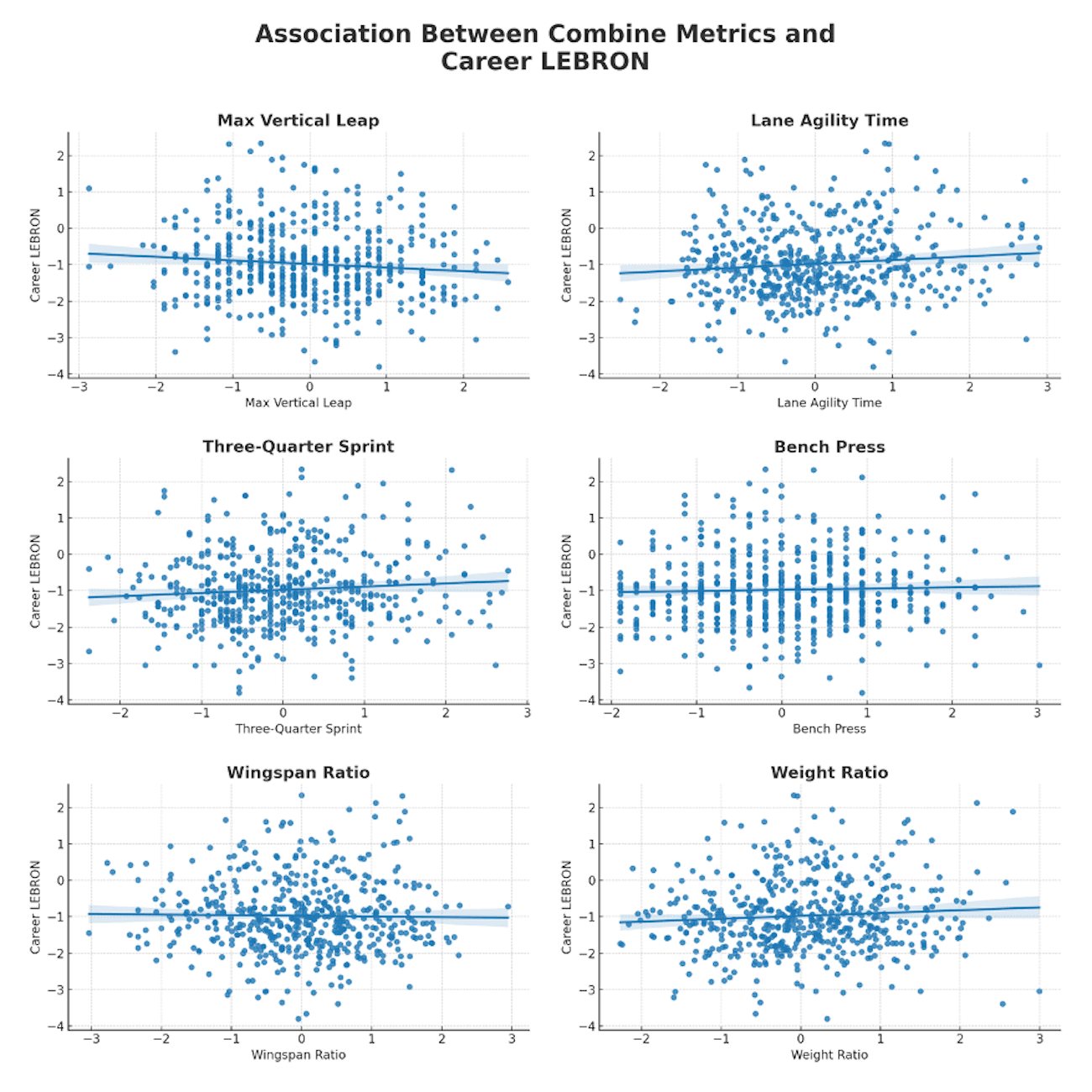
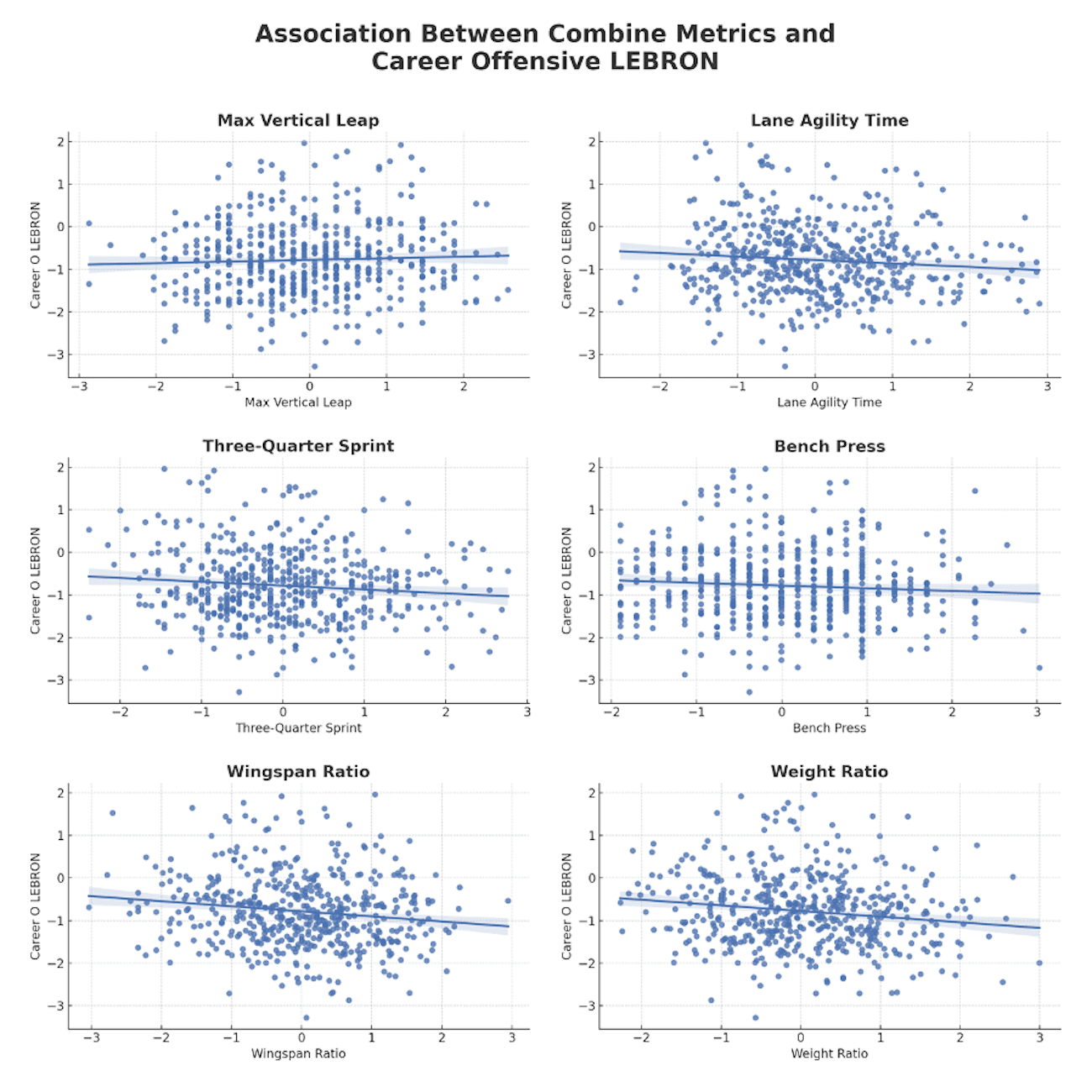
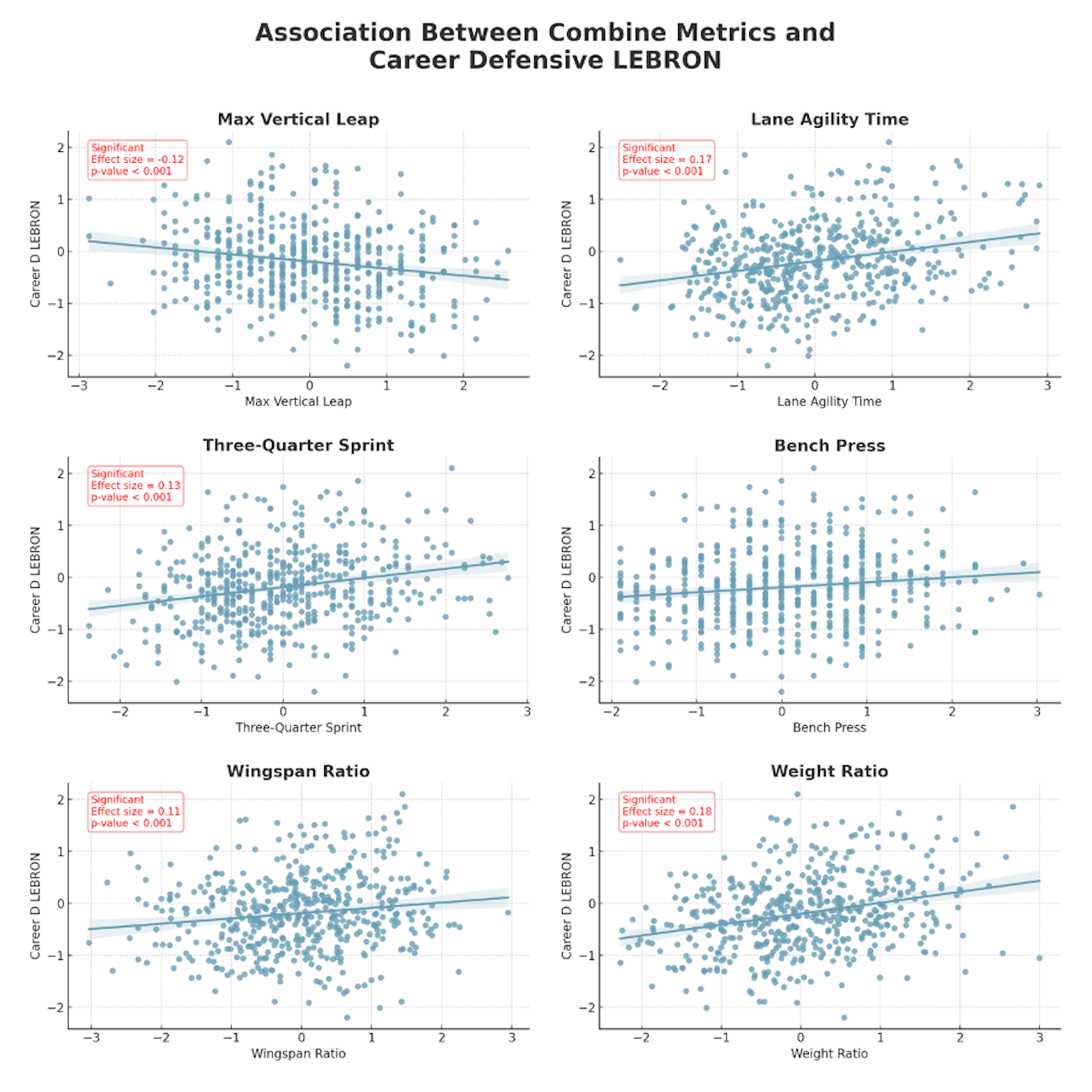
Here’s a summary of the findings:
No metrics were significantly associated with LEBRON or offensive LEBRON.
Defensive LEBRON, on the other hand, was significantly associated with every metric except bench press. The largest association was found for weight ratio, which has an effect size of 0.18. That is, for every standard deviation increase in weight ratio, there was an average increase in D-LEBRON of 0.18.
Surprisingly, we also found a negative association with max vertical leap and positive associations with lane agility time and three-quarter court sprint (i.e., slower times were associated with higher D-LEBRON). Apparently, elite physical anthropometry matters for good defense, but athleticism might actually make you worse.
That last point was as unintuitive to us as it probably is for you, so we decided to dig a bit deeper into that. Specifically, we considered the possibility that there might be interaction effects with position at play here. Maybe speed and jumping ability only matter for guards, while things like bench and wingspan ratio might have an outsized effect for bigs.
The association between NBA draft combine metrics and LEBRON – broken down by position
To see if there were different effects by position, we conducted the same linear regression analyses, but stratified by position.
Here are the same scatterplots as above, this time with separate regression lines for each position alongside the original regression lines representing all positions combined:
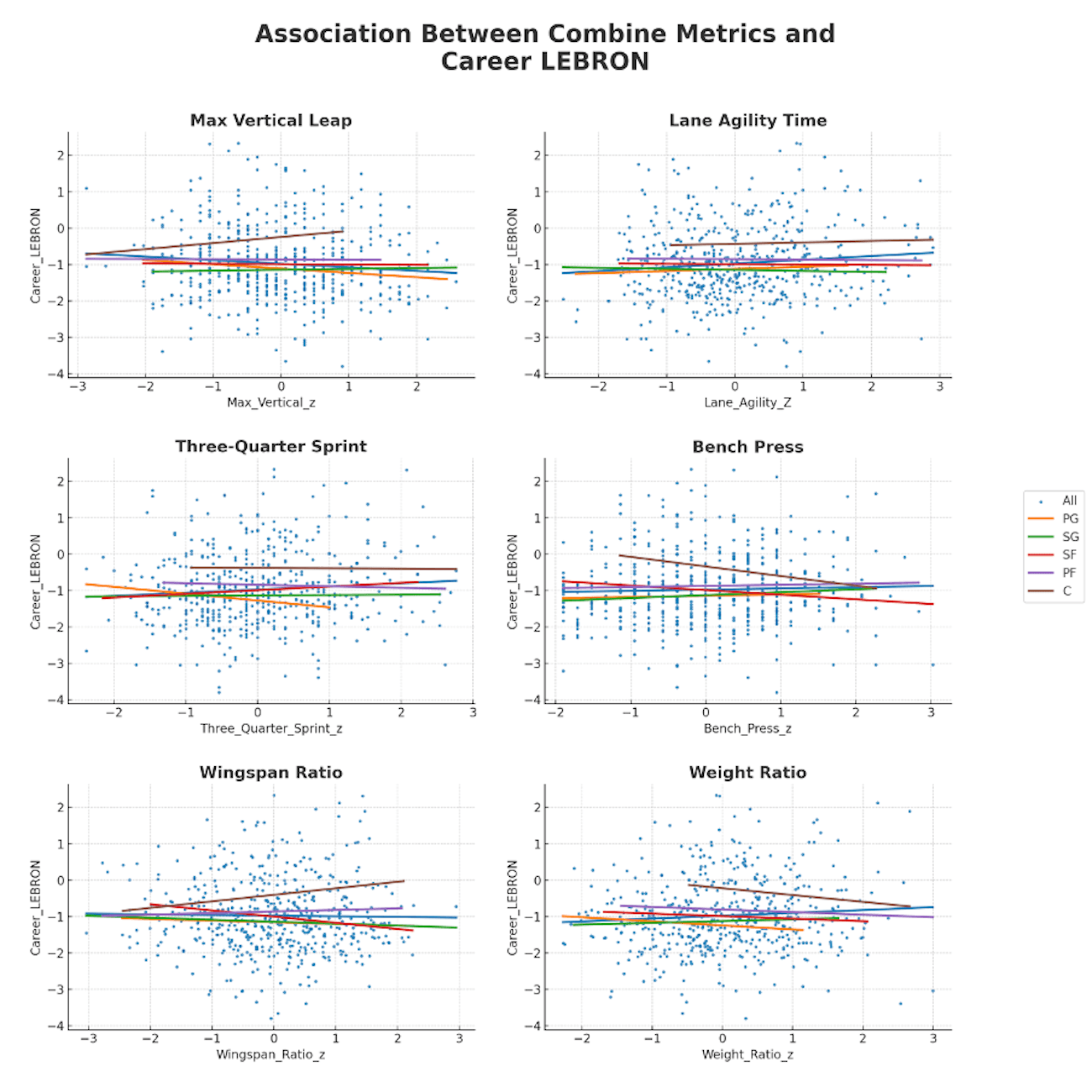
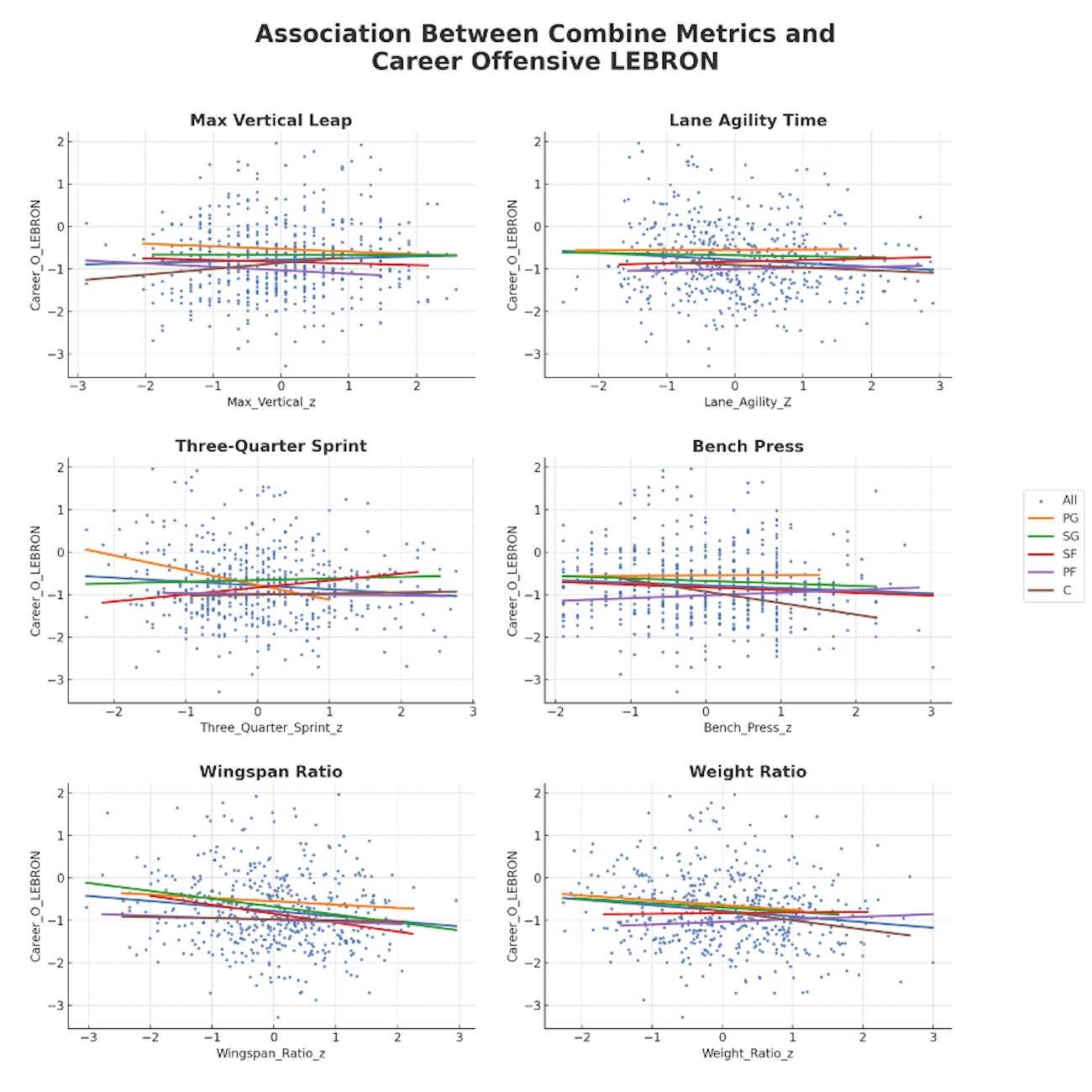
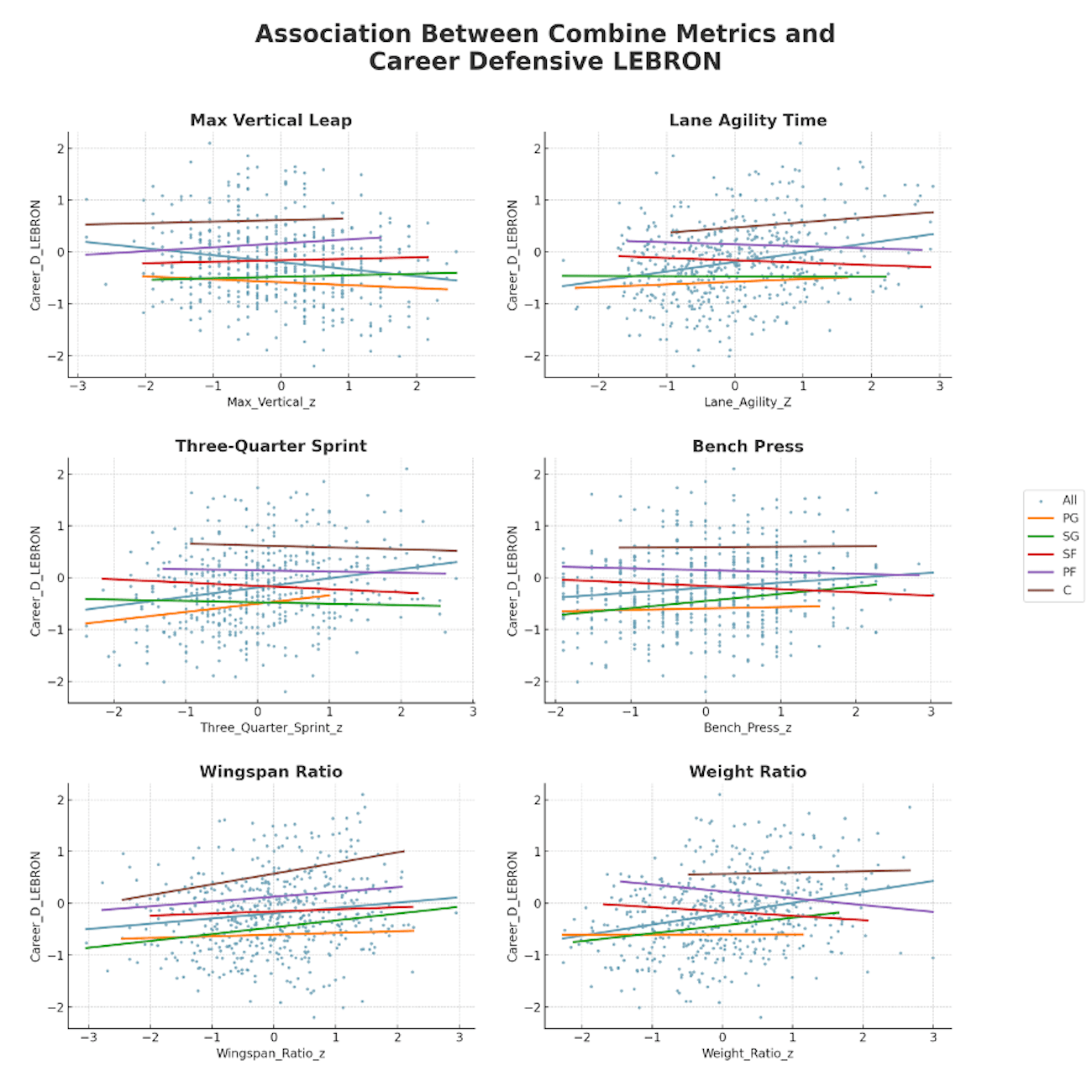
Before we delve into the findings here, we should note that no positional effects were statistically significant after adjusting for multiple comparisons. Since sample sizes were much smaller when breaking things down by position (e.g., there were only 87 centers with wingspan ratio data), we likely lacked the statistical power necessary to detect significant effects.
ELI5 explanation for “adjusting for multiple comparisons”: running so many models at once (multiple comparisons) increases the chances of finding false positive effects, so it’s best practice to set a much stricter threshold for statistical significance when doing so. The downside is that this also increases the chances of false negatives—failures to flag an effect as significant when it really is.
Does that mean there’s nothing meaningful to takeaway from these models? Absolutely not. While we need to be mindful of making extravagant claims with limited statistical power, there are still interesting trends we can observe.
To identify these trends, let’s look at some of the effects that were statistically significant prior to adjusting for multiple comparisons:
Three-quarter court sprint time was negatively associated with both LEBRON and O-LEBRON (i.e., quicker times, higher LEBRON) for point guards only. The effect size for O-LEBRON was the largest in our entire dataset at -0.38.
Wingspan ratio was negatively associated with O-LEBRON but positively associated with D-LEBRON for shooting guards and small forwards.
Wingspan ratio was positively associated with D-LEBRON for power forwards and especially centers. The effect size for centers was 0.14, which was larger than the effect for any other position.
The fact that straight-line speed appears to matter only for point guards seems intuitive. When you’re the smallest guy on the court, you don’t have the privilege of using size, strength, and timing to manipulate defenders a la Luka Doncic; you need to blow past them. It also tracks that the effect wasn’t found on the defensive end, where lateral agility is far more important than straight-line quickness.
The wingspan ratio reverse uno card for shooting guards and small forwards is rather less intuitive. On the defensive end, it makes sense: having long arms as a wing helps with locking up ball-handlers and intercepting passing lanes (Kawhi Leonard comes to mind). On the offensive end, disproportionately long arms... might be disadvantageous, on average, for shooting? The verdict is out on that one.
And finally, there’s wingspan ratio and defensive prowess in bigs. This one might be the most intuitive of them all. In recent memory alone, the long arms of guys like four-time Defensive Player of the Year winner Rudy Gobert and once-in-a-lifetime prodigy Victor Wembanyama have given us plenty of eye-test evidence that wingspan might be the most important attribute of all for interior defenders. There’s nothing like freakishly long arms to alter—or altogether erase—shots from guards who dare to enter the paint.
Here's a closer look at the correlation between wingspan ratio and D-LEBRON in power forwards and centers:
(note: Wembanyama, Jokic and Embiid weren’t actually in our original analyses due to missing NBA combine measurements, but we manually inputted their numbers from data available elsewhere for this graph since they’re three of the biggest names in the sport right now).
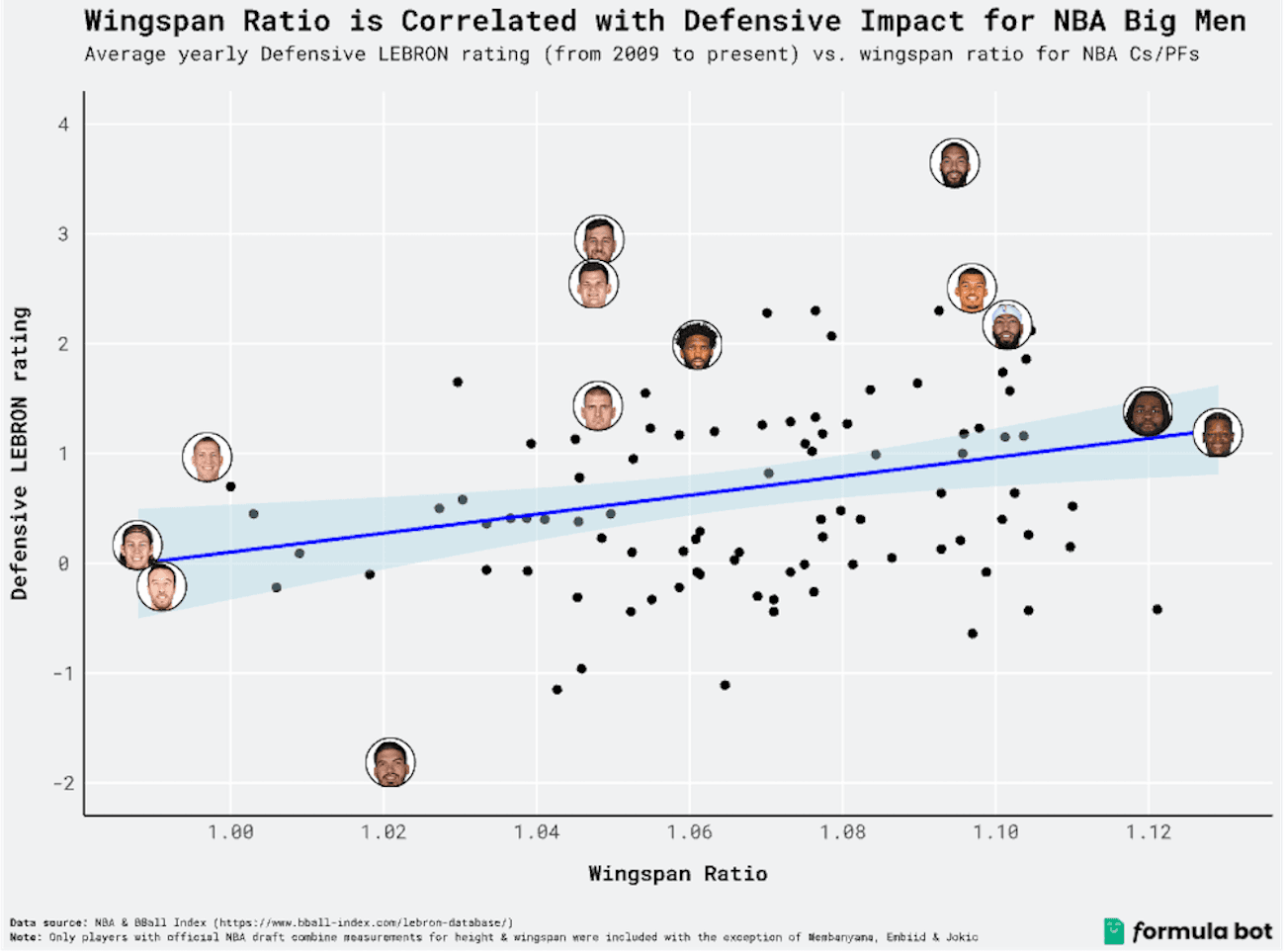
Predicting LEBRON from NBA draft combine metrics: random forest model
Okay, correlations and effect sizes are cool and all, but the question asked in our title was if NBA combine metrics can predict NBA success. After all, executives are gonna be spending a lot of money on these guys—can draft combine metrics help inform business decisions?
To answer this, we decided to run a machine learning algorithm called a random forest model. Instead of analyzing the association between each metric and the outcomes individually, this method allows us to throw all of our metrics into one predictive model.
Under the hood, you can liken a random forest model to asking a bunch of decision-makers (decision trees in technical terms) to each give their opinion about whether certain NBA combine metrics predict success. Each tree looks at the data in its own way, and by combining the results of all the trees, we get a more accurate and reliable answer. Think of it like getting a second (or hundredth!) opinion before making a big decision—more perspectives help reduce the chance of mistakes.
The results from a random forest model give us two key insights. First, we see how well the model works via fit metrics—basically, how good it is at predicting NBA success based on the combine metrics. Second, we get something called feature importance, which tells us which combine metrics are the most useful in making accurate predictions. This helps us understand which measurements matter most when trying to forecast NBA performance.
Let’s first take a look at our fit statistics for our three random forest models (one for each LEBRON outcome):
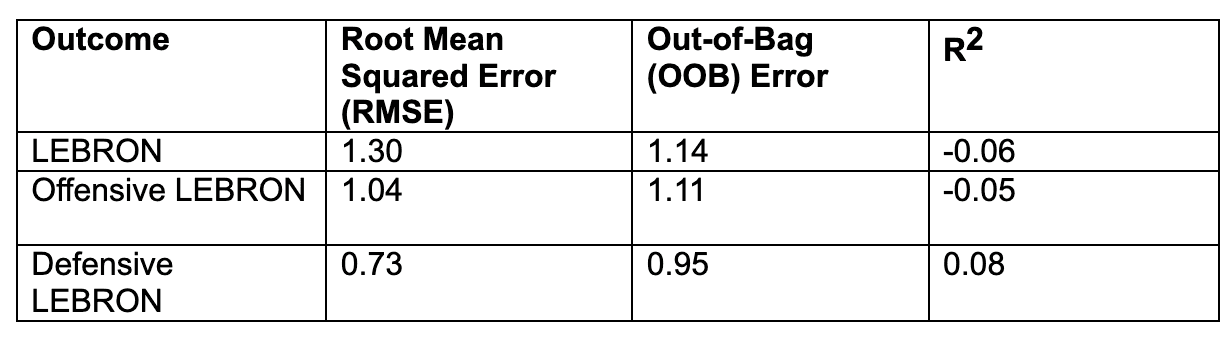
RMSE tells us how far off the model’s predictions are from the actual values. In terms of RMSE, then, the combine metrics most accurately predict Defensive LEBRON.
OOB error tells us how accurately our model predicts the outcome without setting aside training data; lower values are better. In terms of OOB error, the Defensive LEBRON model was again the most accurate.
R2, or variance explained, tells us how well our model explains variation in our outcome; the higher the R2, the more predictive our model is. R2 values should be between 0 and 1, so the fact that the LEBRON and O-LEBRON values are negative tells us that those models are extremely bad fits, and that they aren’t predicting the outcome at all. On the other hand, combine metrics explain around 8% of the variance in D-LEBRON, making it the best fit once again.
Okay, so our combine metrics explain some variance in Defensive LEBRON. But which metrics are the most important? Here are the feature importance values:
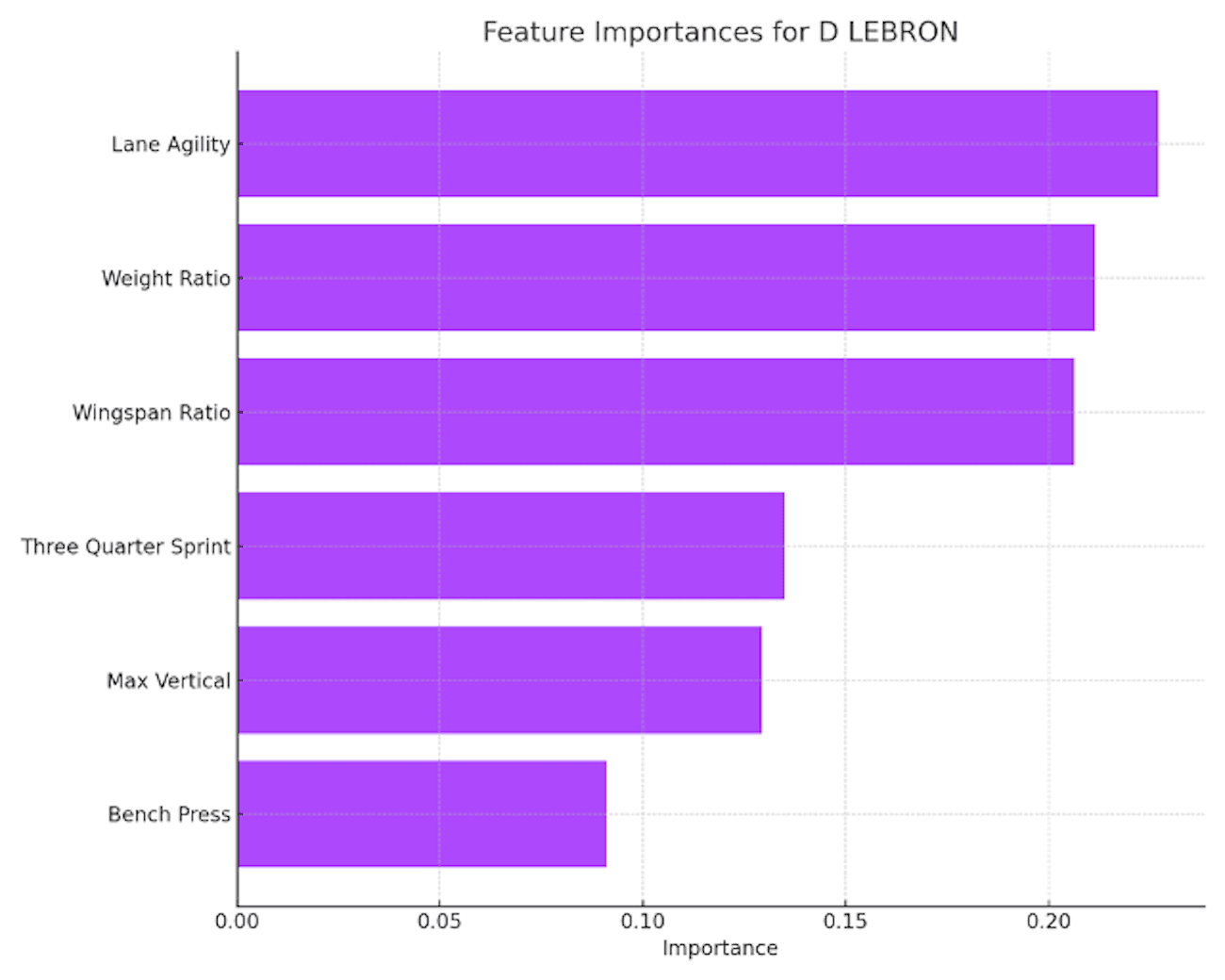
These results corroborate our initial non-stratified regression analyses, with the order of most important to least important metrics almost perfectly aligning with the order of largest to smallest effect sizes in our regressions. Poor bench press.
Takeaways
Alright, so let’s imagine you’re a GM in charge of figuring out who you want to take with your second round pick. What combine metrics are you looking at to influence your decision—if any?
For offense, three-quarter sprint speed is the only metric that might reliably translate to NBA success—but only for point guards.
For defense, all metrics combined provide a little bit of predictive utility, explaining about 8% of the total variance in D-LEBRON.
Looking at the metrics individually, slow lane agility times and a high weight ratio seem to be the most important overall for D-LEBRON, although there are inconsistent effects (some positive, some negative) depending on position.
Wingspan ratio is the only metric with a consistent positive association with D-LEBRON across all positions. The effect is especially pronounced for centers.
Aaaand... that’s pretty much it.
Of course, this isn’t to say that raw athleticism doesn’t matter—it most certainly does. But every player who makes it to the NBA draft combine is already an elite athlete; when there’s such limited variability (at least compared to the general population), it’s no surprise these metrics don’t really offer much predictive value.
So, while combine measurements offer some insight into a prospect’s athletic talent—one part of the overall package—there’s so much more that makes a basketball player great (or even just good). There’s skills, of course, but there’s also the intangibles: the feel for the game, the mental toughness, and, perhaps most important of all, whether or not he’s got that dog in him.
Digital Marketing
Google Search Console vs Google Analytics
Sports
What's happening beneath the surface of the college football transfer portal
Sports
Which college teams are best at preparing players for the NFL? See where your team ranks.
Sports
A Method to the Madness: Machine Learning Reveals Wild March Madness Predictions
Sports